Embracing AI is no longer a choice but necessary for businesses to stay competitive. Rather than resisting this technological shift, companies should proactively harness the potential of AI to drive strategic advantages. Attempting to restrict its use will undoubtedly result in Shadow AI – the use of AI within businesses without formal approval or oversight, potentially leading to security and compliance risks.
When I started this blog, I didn’t want to write about the same things from the same perspective as everyone else. I tried to find new angles to discuss and contribute to the overall body of knowledge, but I have written very little over the last few years. After embracing AI about a year ago, I can focus on AI governance and bring it together with Information Security, Privacy, Risk Management, and Internal Audit.
After completing the ISACA Artificial Intelligence Fundamentals certificate and becoming “Certified in Emerging Technology (CET)”, I decided the next phase would include the new ISO 42001 Lead Auditor and Artificial Intelligence Governance Professional (AIGP) qualifications. I recently developed an AI policy and embarked upon a journey to deliver ISO 42001:2023.
Here are some important considerations:
- Compliance with regulations – Ensure that AI applications adhere to local and international laws, including the General Data Protection Regulation (GDPR), which govern data protection and privacy. Failing to comply could result in severe financial sanctions and reputational damage. Robust data protection measures are essential.
- Ethical AI use – Ethical guidelines are essential to prevent AI from perpetuating bias and discrimination. Businesses should implement working practices that ensure fair, transparent, and explainable AI decisions, allowing stakeholders to understand how outcomes are derived. AI has the potential to become “Computer says NO” on steroids. Transparency is essential.
- Data Quality – The success of AI relies on the quality of data used for training models – garbage in, garbage out. Accurate, relevant, and current data is essential to produce reliable output. Data cleaning and validation practices help maintain data integrity.
- Reliability – AI models must perform reliably across various scenarios. Regularly evaluating and updating models ensures they remain accurate and effective in changing environments, preventing degradation over time.
- Alignment with business objectives – AI initiatives should directly support strategic goals. Identifying specific business problems that AI can solve and setting measurable outcomes ensures that AI investments deliver tangible benefits.
- Employee training and involvement – Providing employees with the necessary training to embrace AI tools helps further develop a collaborative environment and significantly increase productivity. Involving staff from different departments ensures that AI applications meet diverse needs and gain wider acceptance.
- Change management – Implementing AI will require significant changes throughout the business. Effective change management, including clear communication about the benefits and addressing concerns.
- Continuous monitoring – Ongoing monitoring of AI systems is crucial to ensure they continue performing as expected. Establishing feedback mechanisms allows for timely adjustments based on user input and evolving requirements.
- Governance framework – A structured governance framework is critical for overseeing AI initiatives. Clearly defined roles, responsibilities, and accountabilities help manage the lifecycle of AI projects.
- Risk assessment – Conduct thorough AI Impact Assessments (AIIA) and address issues before they escalate to ensure the resilience of AI systems.
- Social Responsibility – Firms should consider the broader impact of AI on society. Using AI for social good and avoiding harm contributes positively and aligns with corporate social responsibility goals. Regularly assessing the ethical implications of AI use is essential to align with societal values.
- Vendor due diligence and technology evaluation – Evaluating the credibility and reliability of AI technology vendors ensures adherence to best practices and ethical standards. Understanding technical capabilities, limitations, and future-proofing allows businesses to adapt without significant rework of existing solutions.
Understanding these considerations will help businesses deal with the complexities of AI integration and ensure the ethical, effective, and sustainable use of AI technologies. Future articles in this series will include:
- Data Privacy Implications
- The EU AI Act and other legislation
- Integration of ISO42001:2023 into ISO27001:2022
- Conducting AI Impact Assessments
My professional goals include becoming a subject matter expert in AI governance to accompany my existing ISACA qualifications – Certified Information Security Manager (CISM), Certified Information Systems Auditor (CISA), and Certified in Risk and Information Systems Control (CRISC).
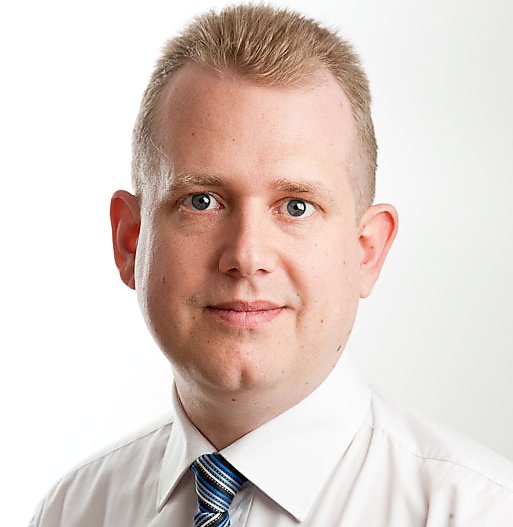
Information security, risk management, internal audit, and governance professional with over 25 years of post-graduate experience gained across a diverse range of private and public sector projects in banking, insurance, telecommunications, health services, charities and more, both in the UK and internationally – MORE